Episode 4: What Makes Data Good for AI?
In this episode of BizTech Forward, we chat with Yuri Gubin, Chief Innovation Officer at DataArt, about why data quality is critical for AI success. From real-life analogies — like cooking and road trips — to the risks of bias and the future of AI learning from AI, we break down complex topics in a lighter way. Tune in for an insightful conversation!
Transcript
Anni Tabagua: Welcome to the new episode of BizTech Forward! The podcast where we delve into the wealth of technology and business with some of the brightest minds in DataArt. I'm Anni from the Media Relations team, and I get to work with these brightest minds every day. So, think of me as your friendly tour guide as we discuss the past, present, and future of tech.
Today, we are discussing a hot topic: data quality in AI. We will answer the big question: What makes data good for AI, and how can bad data totally mess things up? To help us unpack all of this, we are joined by Yuri Gubin, Chief Innovation Officer at DataArt. Welcome, Yuri.
Yuri Gubin: Hi, Anni.
Anni Tabagua: Just a brief intro. As I said, Yuri is the Chief Innovation Officer at DataArt, and he helps clients solve complex technology problems and rebuild their businesses. He also leads many labs at DataArt, like AI, DevOps, cloud solution architect board, and many partnerships. Yuri's a technologist and a mentor, among many other things. So, it's such a pleasure to have you with us, Yuri.
Yuri Gubin: Yeah, thank you. You're absolutely right about data quality and AI, what it entails, where things are going, what the expectations are, and what the pitfalls are. I think everybody needs to be concerned about this right now and moving into 2025.
Anni Tabagua: Right. And before we dive in, just a quick note. We usually break the show into three fun parts: past, present, and future. And we always sneak in a little unpopular opinion from our guests. So do not miss that. On that note, let's get right to it. In the past, how did we get here? So, let's start by going back in time a little bit.
Data is surely essential for the success of any artificial intelligence project. Data and AI have been linked forever. But was data quality always a top priority? Was it always this big of a deal? So basically, what was it like in the early days?
Yuri Gubin: Yeah. So, data quality — and don't get me wrong, data quality was, is, and will be paramount in data management. It is crucial to have good data to make decisions and to consider the trajectories and trends that drive the business and strategy. But the thing that changed is that the context we are talking about, about data quality, is AI.
In the past, the trajectory was that companies grew this AI capability, and it depended on a very strong data foundation. By doing the data right, you naturally grow into advanced analytics and machine learning. But what changed in the last 18 to 24 months is that AI is now very accessible. It is very powerful, generative, and can handle natural language structure.
It can handle everything. And it is right there. There is an API, there is an interface. And you can start building your AI applications. So, even without a strong data foundation, everybody jumped into AI and the adoption of AI. What has really changed is that you see the implications of poor data quality for your products and your applications.
And the setting where we are right now is that we really talk about poor data quality and how it affects AI when really we should not have this conversation because it's so essential to have the data right, the infrastructure, the ownership of the data, and many other things to do AI the right way. Yeah.
To be more precise, with AI models in general, garbage in, garbage out. Whatever data you feed it, it will generate insights, advise on decisions, or generate text based on what you give to it. Overfitted models, decision biases, poor quality, and accuracy of responses all stem from either your data or data that was used to train the original model. When we talk about biases in AI, we need to talk about biases in data that we have accumulated or used to train that model. So it's much more complex than just pointing to, say, data quality or missing fields or duplicates or incompleteness of the data and pointing that, yeah, this is why we have poor AI, no, it's a much bigger picture.
Anni Tabagua: I guess I'm oversimplifying a little bit, but to bring it down more to an everyday example—and I don't know — would it be fair to compare this a little bit to cooking? Maybe I'm one of the world's best chefs, and I only have really bad ingredients.
Yuri Gubin: You're absolutely right. This is a very good example. No matter how skilled you are as a chef, it will be very difficult for you to cook something with poor ingredients. And yeah, you're spot on. I have another example. It happened in the past in healthcare, from medical research to the root cause analysis of what was causing the ulcers.
You know, medical conditions in the stomach. For years, the perceived reason for that was that stress was causing it. So when you analyze even the image, the data set. And every patient with an ulcer has stress. So if you only have that data, then probably yes, you will. And a machine learning model will tell you that stress is the reason it caused the ulcer.
But we now know that bacteria cause it. So, the stress in that equation was not the cause; it was just a correlation. And that's a mismatch.
Anni Tabagua: Oh, wow. That is a serious mismatch.
Yuri Gubin: Yeah, it is. It is important to have complete data. And this is real-world evidence of why we need to pay attention to data quality.
Anni Tabagua: So, this brings us to now, to the present. Which is like, I don't know, probably the most important thing and something to pay attention to, but what is it that's happening now? Exactly. So what you keep talking about is the data quality and how crucial it is to get it right, but how we can get it right? How are companies ensuring that their data is up to the task today?
Yuri Gubin: Yeah, it's a good question. So, aside from having the technology to handle the data, yes, you think about the methodology and data management practices, and you integrate all the checks and controls that will just enforce good data quality. We talk about introducing a framework of data stewardship, data mesh, data ownership, and data as a product.
It's when you have stakeholders and subject matter experts in your team who understand the data. It's natural to see something beyond just, you know, tables and namespaces in a database, someone who understands the nature of the data. This is very important because, you know, the person who is very good with models, wrangling the data, and crunching the data sets might not be the best person to understand the business.
What it means for the business to have this data, what it means to interpret, you know, how to make an interpretation from an insight that a machine learning model is producing. That's why we need data stewardship. That's why we need SMEs to actually work side by side with data scientists. One of the examples that I was thinking about is if you look at the tech, you have parents, teachers, and students.
If you group them together in one table as users of your online LMS and then start crunching that data set, you will be comparing people who should not be compared to each other. That's why the person who understands the nature of the data says, "All right, this adult is a parent."
It should not be treated like a teacher, facilitator, or supervisor. That person can actually divide the data set into more granular and appropriate buckets.
Anni Tabagua: Oh, that's actually a bit surprising for me to hear because since it's all about the tech lately, it's all we think about. I almost forgot that we might still need people. And that's exactly the case, right? Which is what you call data stewardship. And that means it's not just about the big database and the technology side of it. It's actually very important to have the people there who know what the data really means.
Yuri Gubin: Yes, it is. It's super important because it's not another system that is using your product. You're doing something for people at the end of the day, and yeah, even if you're in the B2B space, there will be end consumers who will appreciate everything that you are doing. That's why SMEs' involvement is very important.
And decisions that AI is making — yes, sometimes — affect real people. That's why people need to be involved in decision-making, too.
Anni Tabagua: And that's so interesting. Yuri, I'm curious to know maybe one more example I can apply to my life. So, I keep thinking of how this affects me. Let's say, for instance, I use Google Maps a lot.
Yuri Gubin: Yeah. So you are in an EV car, right? And you want to charge it now. You point to the next charger, and your map tells you how to drive there. Technically, you know that there are different highways, and on certain highways, there is a middle lane that you cannot cross.
It can be a physical barrier. So imagine the situation when you arrive at the EV charger place on the map, but you realize that the station is on the other side of the highway. So, to get there, you have to actually make another U-turn. And it can be, in some cases, a lengthy journey. But yeah, you need to expect this from your map to understand the rules of navigating the highway, what it means to make a left turn here or a right turn there, and where the things and businesses are located. Aside from just having roads in your memory.
Anni Tabagua: And this is where the people come in.
Yuri Gubin: Yeah, this is where the people can come in because someone needs to explain the rule that you cannot make this turn here.
Anni Tabagua: Okay, now I understand a bit better. You mentioned right at the beginning how important bias is in the context. I wonder if you can talk a little bit more about that because I see a lot of people talk about bias in AI and how companies are addressing that today. How important is that?
Yuri Gubin: Yeah. So, I want to start by saying that things have changed. It's not the companies that are primarily concerned with AI; it's the regulation that is concerned with it. Right here in the US, we can see that different states are adopting regulations. There are new bills and acts that regulate how you use AI in decision-making, such as hiring or firing people, issuing a driver's license, or benefit distribution.
You must record what you are collecting and the data you are collecting. You must get the consent from the person. You also have to make sure that the model and the process are auditable and report what data you have collected and what decisions were made. Because nobody wants to automate discrimination. Nobody wants to make this an abnormality.
When AI just discriminates left and right because of the data that was used to train this model. So, for example, things that stem from individual characteristics, decisions made around people's age or gender or race, whether people have children, or what about jobs? What about income? This must be taken very carefully because certain data parameters and data fields cannot be used to make decisions. Otherwise, it will be perceived as discrimination. It will be difficult to defend the model because it's a tough problem to develop an explainable AI that will actually explain why it was doing certain things.
Now, there is another example. Nowadays, we talk about denial, and bias, by definition, is disproportionate weight in decision-making when you are disproportionately in favor or against something because of unrelated things. So, with GPT, one of our colleagues pointed out that there is the verb "to delve." It is less used in the US and the UK as a business language and is more used in different countries and other parts of the world. So because of reinforced learning, that word was introduced, and statistically, it is much higher to see this word in the text produced with GPT-3, with certain models.
The bottom line of this is the source bias in the way you train a model in the text that you used to train the model, which will result in the way the model generates content, and then users will actually see that bias. For example, the same word to delve. If you read an article with "to delve" into its title, you may now think it was generated with GPT.
You understand this because it was trained in a certain language in a certain way, by certain methods. You now see all this text has this because it was generated this way.
Anni Tabagua: You're right. This is a good example because it makes me realize that I use that word often.
Yuri Gubin: Interesting.
Anni Tabagua: I'll stop! Yeah, wow. That's good to know. Yuri, since we're still here in the present, I wonder if you have, let's say, an unpopular opinion about data in AI, something that is happening right now that might qualify as an unpopular opinion of your own.
Yuri Gubin: Yeah, I think that models will get better and better. Every other week, there will be a new breakthrough in AI. But will it actually solve the problem? Will it actually do what people want it to do? We don't know. So, instead of focusing on new great things on the market in AI, I would focus on what it should do.
Instead of opting to share more data with AI and integrate new models, I would stick to the basics, think about the product concepts and how I want to develop certain things, and limit the data that I want to be exposed to AI to be more disciplined and have more controls.
You just don't want to throw a lot of good stuff in a can and assume that something good will happen. You need to think about this and own and control this process.
Anni Tabagua: So quality over quantity.
Yuri Gubin: Yeah. Again, yes. Quality is much more important.
Anni Tabagua: I also want to have a whole list of all the words that I should avoid, but I'll talk about it some other time. Yuri, this brings me to potentially my favorite part, which is predicting the future. So, where are we going with all of this? Where do you think AI and data quality are heading? What challenges are we going to face more and more as AI becomes more advanced? These are just some thoughts that you might have.
Yuri Gubin: Yeah, one thing on my mind is the concept of actionable AI, when you can not only just read and make AI write or summarize something for you, but when you can have an engine that has such good quality and when hallucinations and all of these things are by design excluded from its functionality like it cannot hallucinate, it is very prescriptive in certain ways that you can actually work with this AI assistant to actually do something. Book a trip and schedule an appointment, even with a system that the AI engine never worked with.
Imagine that you found a movie theater and want to watch that film. You ask your AI assistant to book that, to buy a ticket for you. And although that AI engine never worked with that provider, never worked with that website, it will figure out how to do it. And you will receive a confirmation email in your inbox. So, actionable AI is something you can trust will not hallucinate in the middle and will actually do something you want for the money you pay, the right place, and the right time. This is something that we need to be looking forward to.
Anni Tabagua: And how realistic is this to get it right, how do you think?
Yuri Gubin: Well, it's tough. Now, we are getting closer to it by means of identifiers, introducing guardrails, and building hybrids between AI and conventional models and workflow automation. But the technology—I keep hearing that technology is not there right now for complex cases—probably needs another round of innovation.
Anni Tabagua: And anything else, like in terms of the challenges around data quality itself?
Yuri Gubin: Yes. What is missing is a data entitlement and built-in data security when every field, every column, and everything is annotated and classified automatically, and models understand that. From the data quality perspective and data privacy perspective, there is a gap that you have to superficially orchestrate. Instead, it should be built into products or platforms, and models should be absolutely respectful of the data entitlements and that mechanism. We all want to break silos in data, but by breaking silos, we should not create new, more complex problems by accidentally leaking data or providing access to certain. So, I think data entitlement is a new opportunity from a data quality standpoint.
Anni Tabagua: I think, you know, I really want to leave our listeners with this wrap-up question. I'm curious: Is there something on the horizon of AI and data right now that you personally are following or are excited about?
Yuri Gubin: It's a very good question. Again, I spend a lot of time thinking about, reading about, and researching actionable AI and its use cases. So that's back to this. Now, this is on my mind: how to remove all the hallucinations and make them predictable. And can we do this right now with our models, like GPT-3, or not? This is something that I'm very curious about.
Anni Tabagua: Okay. I hope we discuss this again next year, and you will tell me more. This has been such an insightful chat, Yuri. Thank you so much. We have covered the importance of data quality and touched upon where we are now and where we're going, and I really loved your examples. I will remember garbage in, garbage out, quality over quantity, and pay attention to the words that you choose because they might point to something.
Yeah, thank you so much. These are definitely exciting times. Thank you for helping us make sense of all that today.
Yuri Gubin: Thank you for inviting me. Thank you for your time.
Anni Tabagua: Thank you so much to our listeners for tuning in. If you enjoyed this episode, don't forget to subscribe, like, and share. As always, we want to hear from you.
If you have thoughts, questions, insights, or opinions of your own, please reach out to us at biztechforward@dataart.com. Thanks again, and until next time.
Yuri Gubin: Cheers.
About the Guest
Yuri Gubin, who spearheads DataArt’s drive toward innovation, is a solutions consultant with more than 15 years of professional experience across the financial services, healthcare, travel and IoT industries. After joining DataArt as a software architect in 2008, he became a leading member of the Solution Architect Board and the Cloud and DevOps center of competence.
Yuri was promoted to his current position of CIO in 2021. He focuses on guiding and promoting several centers of competence and excellence. His role also includes identifying technology trends, cementing alliances and strategic partnerships with other companies, and coaching and mentoring new talent.
Passionate about technology and the latest technological innovations, Yuri is a proven expert in designing solutions that best use big data, AI, DevOps, IoT, ML/AI, cloud, and other technologies. Yuri is certified by both AWS and Google Cloud Platform as a cloud specialist.
We Want to Hear From You!
Reach out to us with any comments, feedback, and questions by filling out the form.
Thank you for contacting us!
We will be in touch shortly to continue the conversation.
Check Out All of Our Episodes
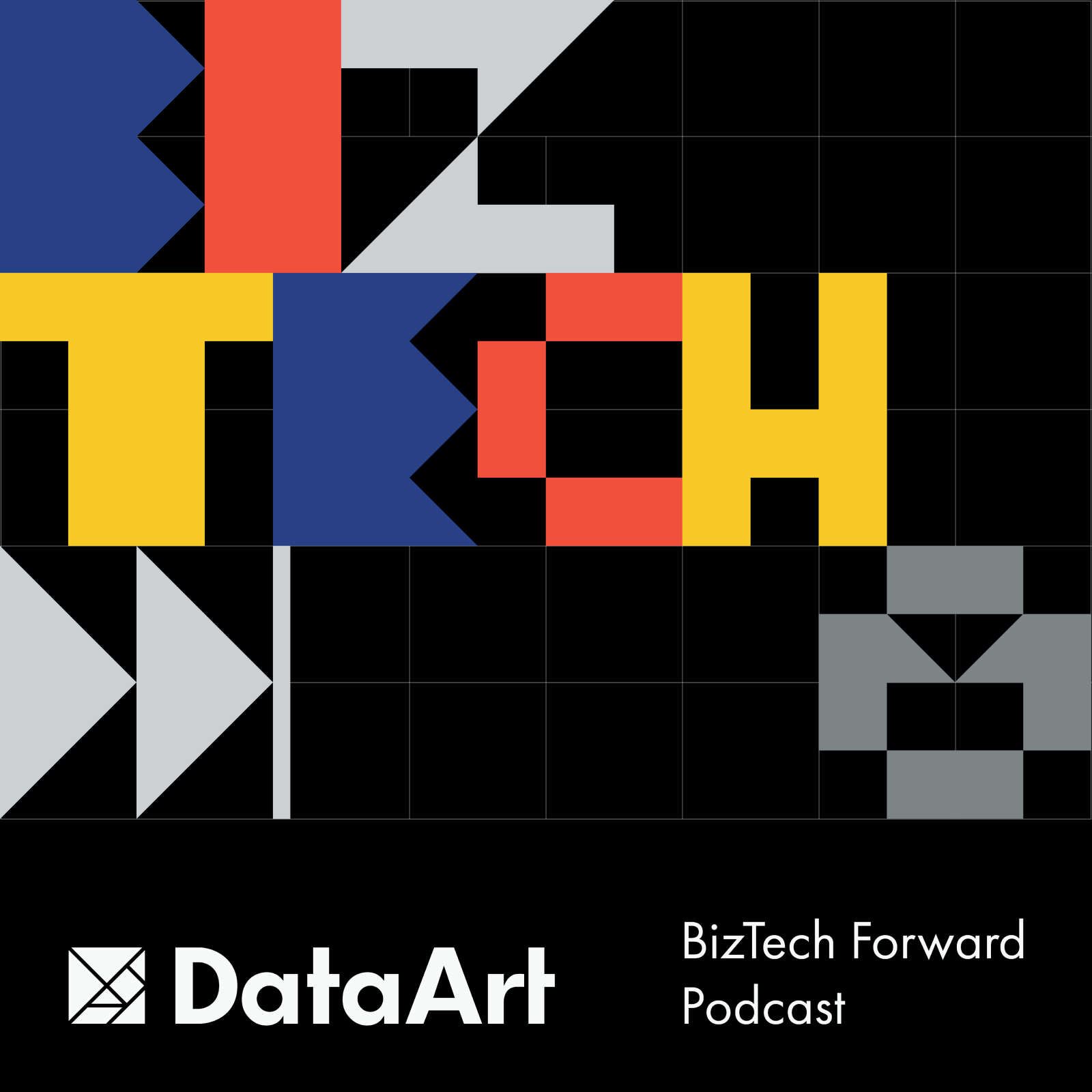
In this episode of BizTech Forward, Anni chats with Scott Rayburn, VP Marketing at DataArt, about how marketing has evolved with the rise of data and technology.
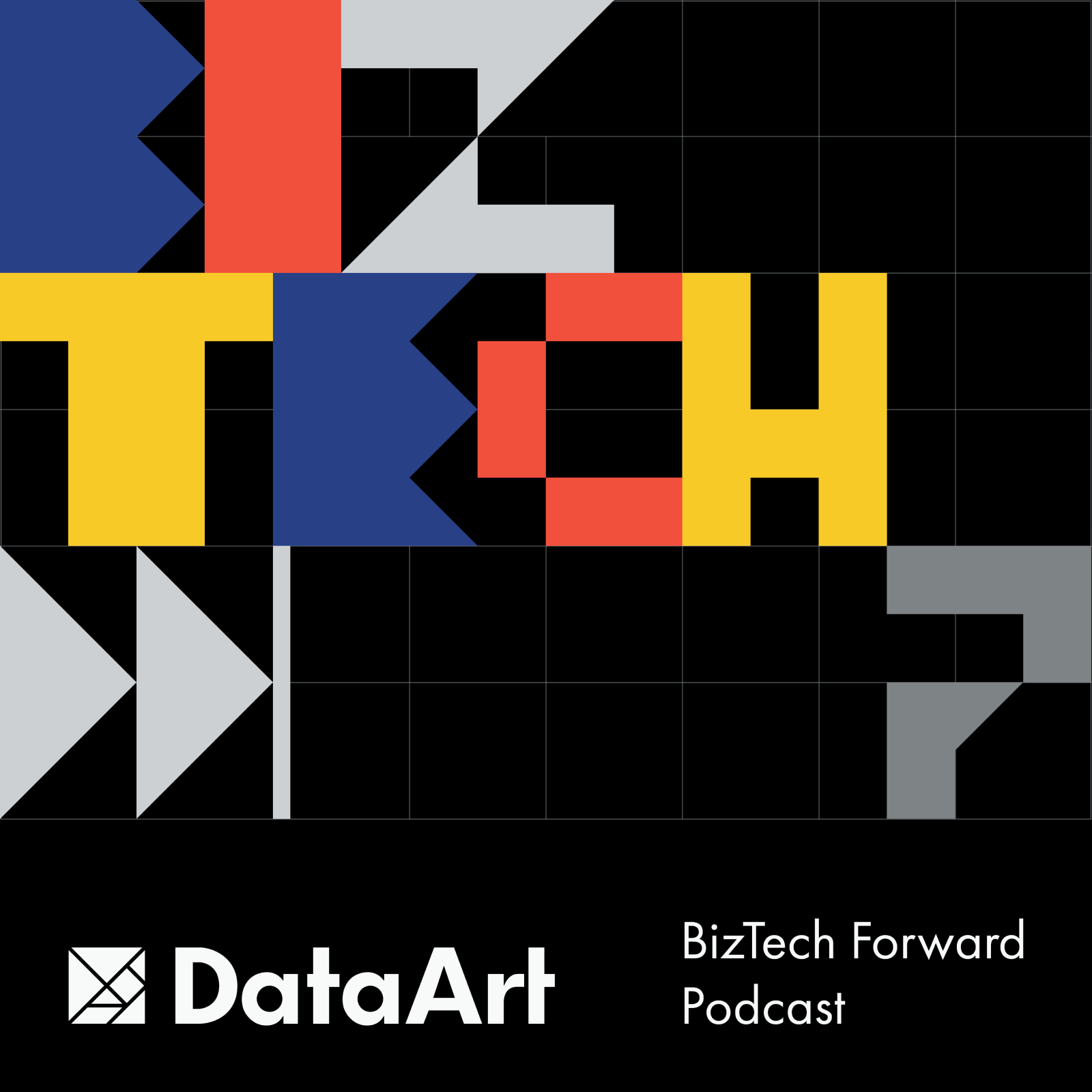
In this episode, Anni chats with Sheetal Kale, Head of DataArt India, about the country’s modern tech views, AI and data, IPO boom, and India’s gravitational pull in global decision-making.
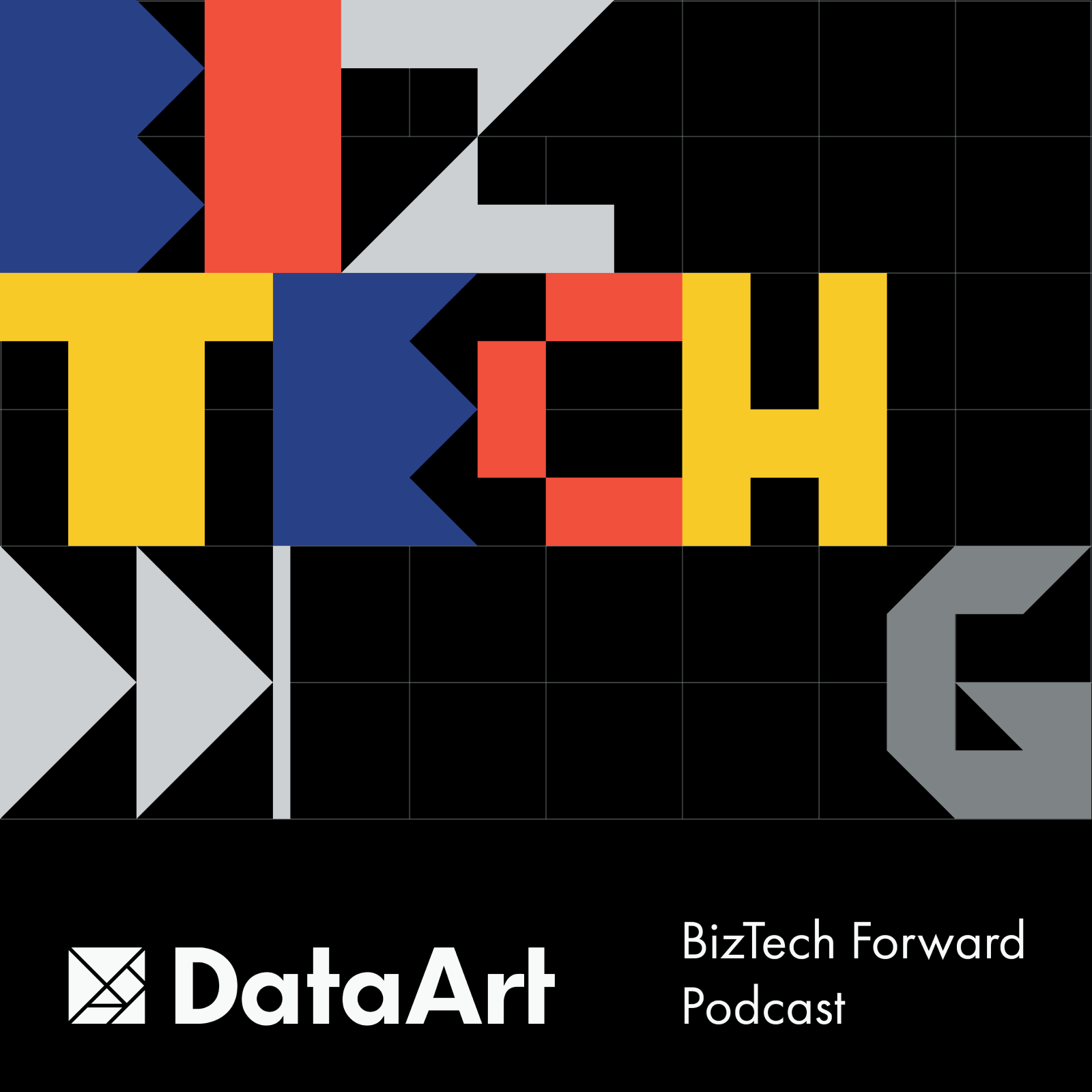
In this episode of BizTech Forward, Anni sits down with Tim McMullen, DataArt's Head of Design Studio, to talk about the evolution of design processes in the world of UX.
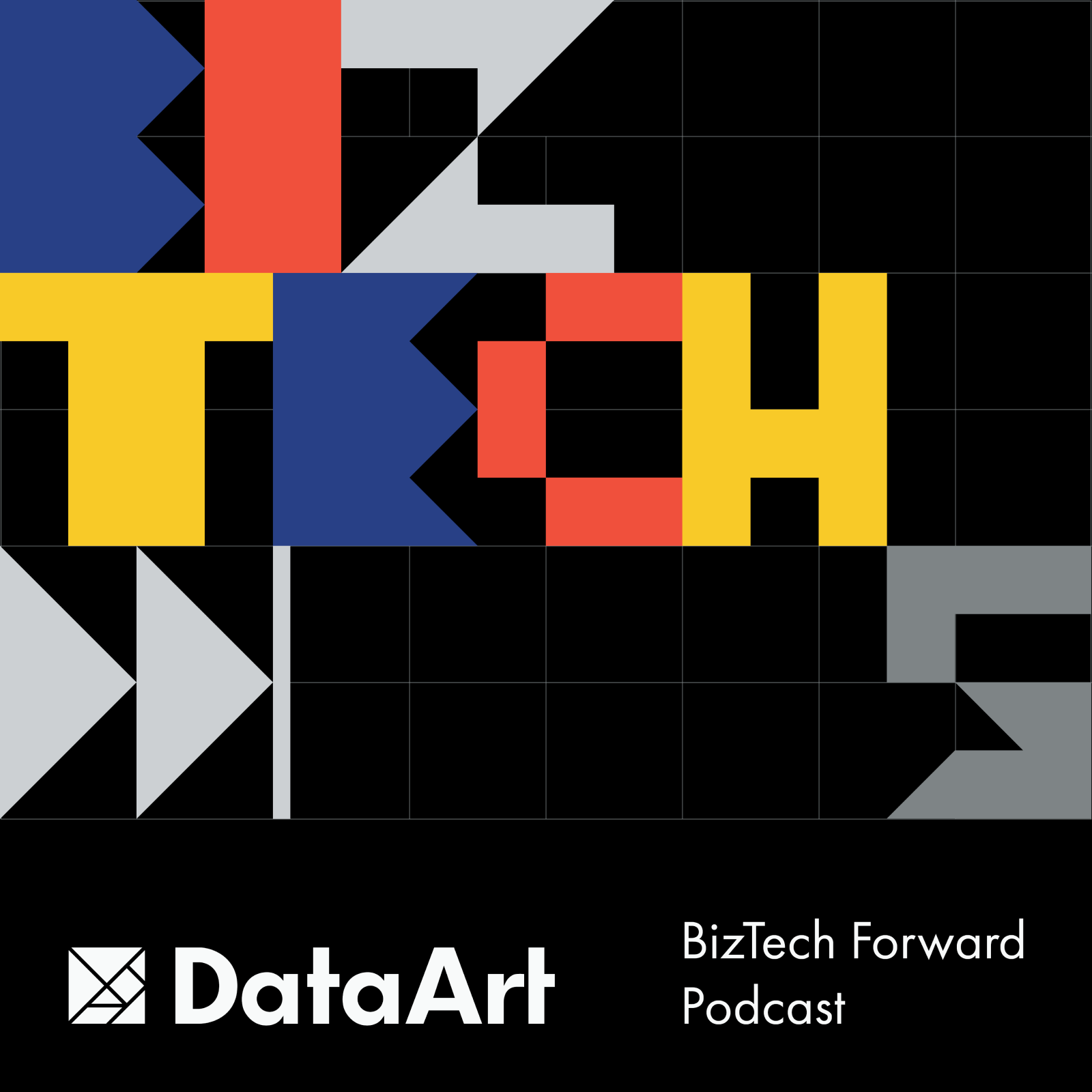
In this episode of BizTech Forward, Anni sits down with Tim McMullen, DataArt's Head of Design Studio, to talk about the evolution of design processes in the world of UX.
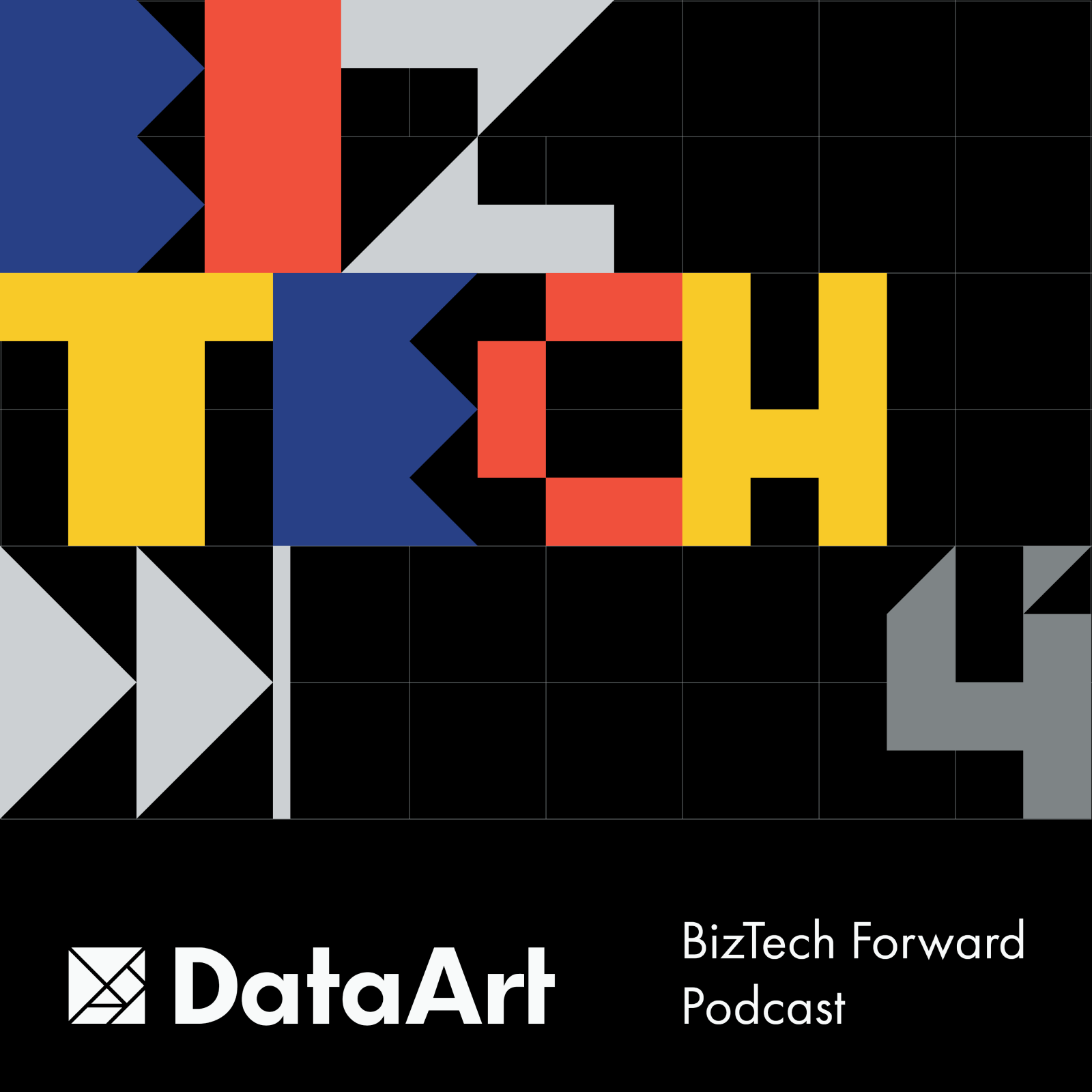
In this episode of BizTech Forward, we chat with Yuri Gubin, Chief Innovation Officer at DataArt, about why data quality is critical for AI success.
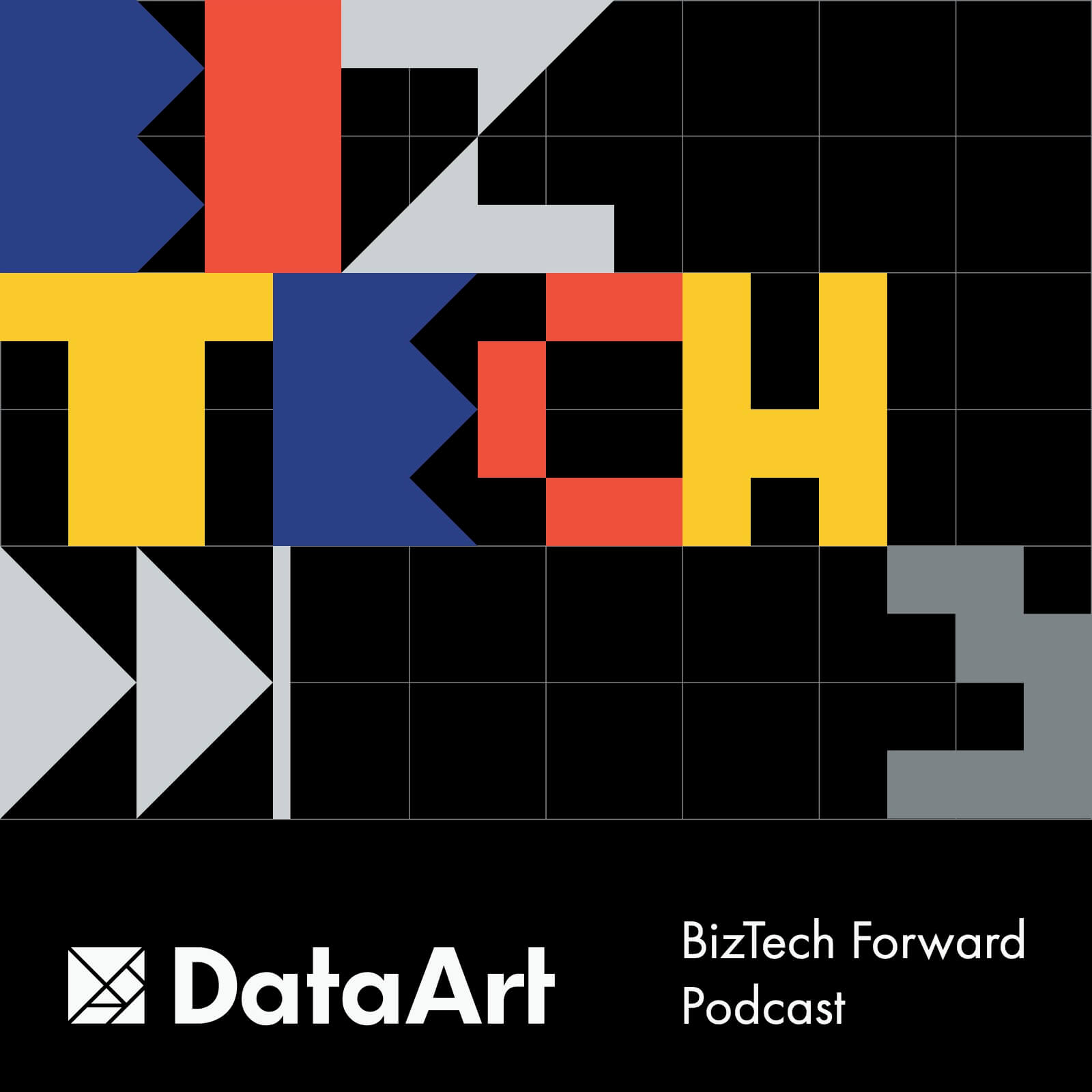
In this episode, we chat with Anna Velykoivanenko, Global Employer Branding Director at DataArt, about the perfect blend of technical know-how and human-centric skills.
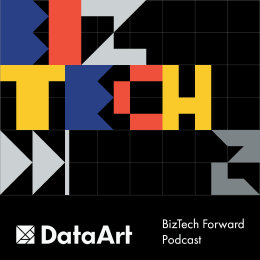
In this episode of BizTech Forward, Anni from DataArt’s Media Relations team chats with Alexei Miller, Managing Director at DataArt, about how businesses can truly measure the value of their IT investments.
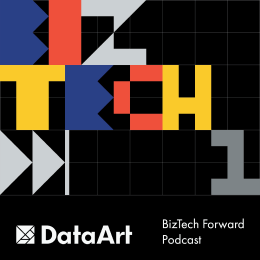
Join Anni Tabagua as we kick off our very first episode with a fascinating topic: AI in Automotive. Our guest is Dmitry Bagrov, the Managing Director of DataArt UK!